Emerging technologies such as advanced analytics and artificial intelligence (AI) are transforming the manufacturing sector. The factory floor is awash with data driven by the growth in Internet of Things (IoT) sensors. But, says Mike Loughran, CTO for the UK and Ireland at Rockwell Automation, data alone is not a useful commodity. This data needs context and domain expertise applied to it before it is analysed to deliver valuable business insights and value.
Analytics and AI has disrupted many industries, especially the consumer space. Today we see targeted advertising and social media ecommerce platforms that can predict the products we want to buy, and location-based apps can even make recommendations based on where you are. The underlying theme here is that analytics allows data driven decisions by surfacing insights at the right time.
Clear benefits
The natural question is how the industrial manufacturing industry, which is quite different from the consumer space, capitalises on this opportunity. The benefits are clear to see. On average manufacturing organisations that are embarking on transforming their operations by adopting digital transformation and analytics are aiming to increase revenue by up to 10%, decrease operating costs by up to 12% and improve asset efficiency by up to 30%.
Other digital technologies are driving this kind of double-digit growth, but when manufacturers try to apply these technologies to analytics, they do encounter some unique challenges. The reason for that is application of analytics in the manufacturing context is complex. Very often analytics is positioned as a turnkey solution where you first gather all the data centrally, and then just apply an algorithm or model to get to the promised land.
Well, it is not that simple. Most industrial analytic workloads probably should not be run in the cloud, due to high network bandwidth costs and longer latency. It makes more sense to deploy those analytic models closer to the edge, where the data is produced. It also takes a lot of work to train an analytic model for an industrial setting. To understand that we need to dive a little deeper into the world of industrial data.
Managing high data volumes
First, manufacturers must manage a remarkably high volume of data that is generated by plant systems in real time, along with historian data. The irony is that, depending on the use case, only a fraction of that mined data might be relevant. They then must integrate this data from disparate sources that might be using different protocols.
These heterogeneous systems might have different legacy technologies as well that might make connectivity and data aggregation difficult. Also, they might not have a common data model in place between systems making the relations, or relationship between data points, rather unclear.
The insights must also be delivered to the relevant person or the system to drive action within a short timeframe to make it relevant. Lastly, applying analytics requires deep knowledge of the underlying industrial processes. It is usually exceedingly difficult to find the data science and process expertise in the same person.
To be successful, it is critical to have a partner who can not only understand both manufacturing and analytics but can also tailor a solution to your use cases. Ideally this partner must have a strong heritage in manufacturing and be familiar with the process hardware and operation technology, and of course your business objectives.
Simplifying data science in practice
What is required are tools to enable the control and process engineers to perform analytics without recourse to data scientists. We need to simplify the practice of data science. When we talk to customers who are on their digital transformation journey, there are two common requirements. The first one is the digital worker and the second is machine learning.
There are four steps that companies need to take with data analysis. First, they must identify the important operational attributes. They can then move on to establish logical data structures. With this achieved they can put practices in place to capture data at high velocity. Finally, there is the need to reuse models across the information layer for greater efficiency and speed. The aim is to accelerate results by equipping engineers with data science tools.
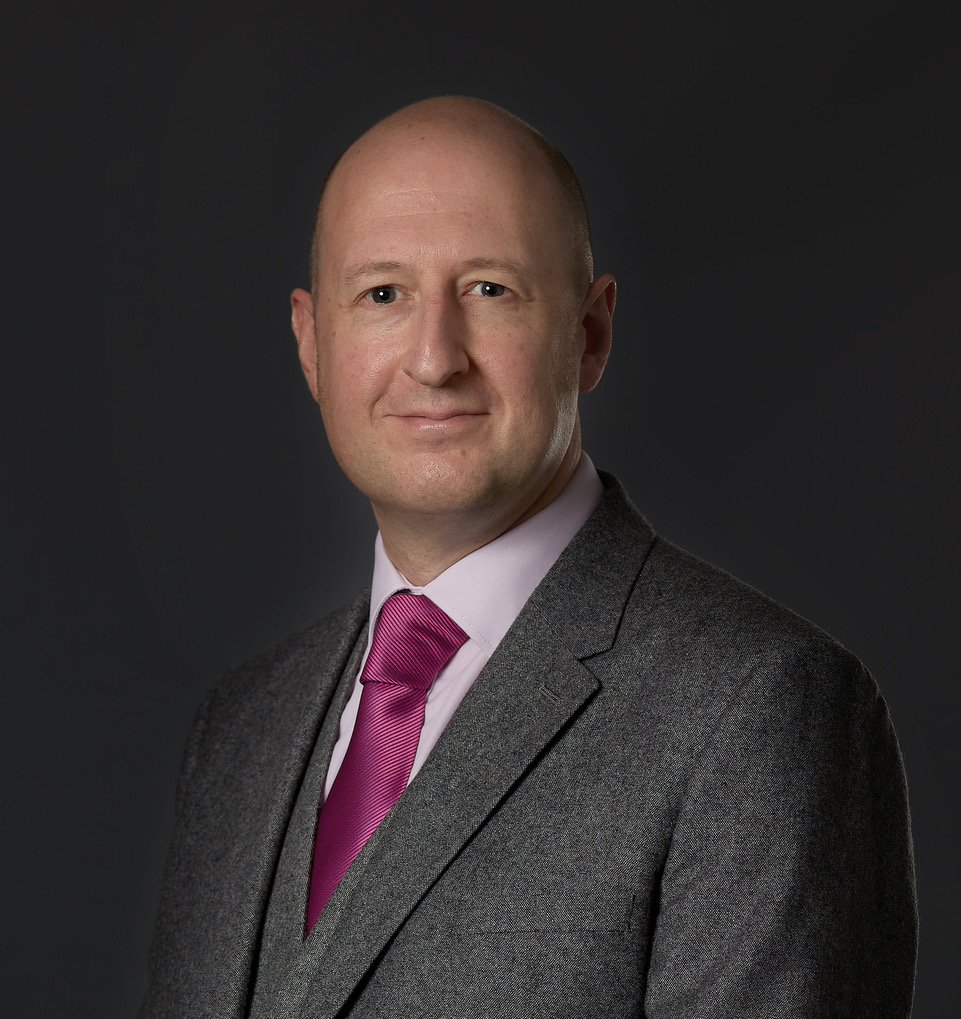
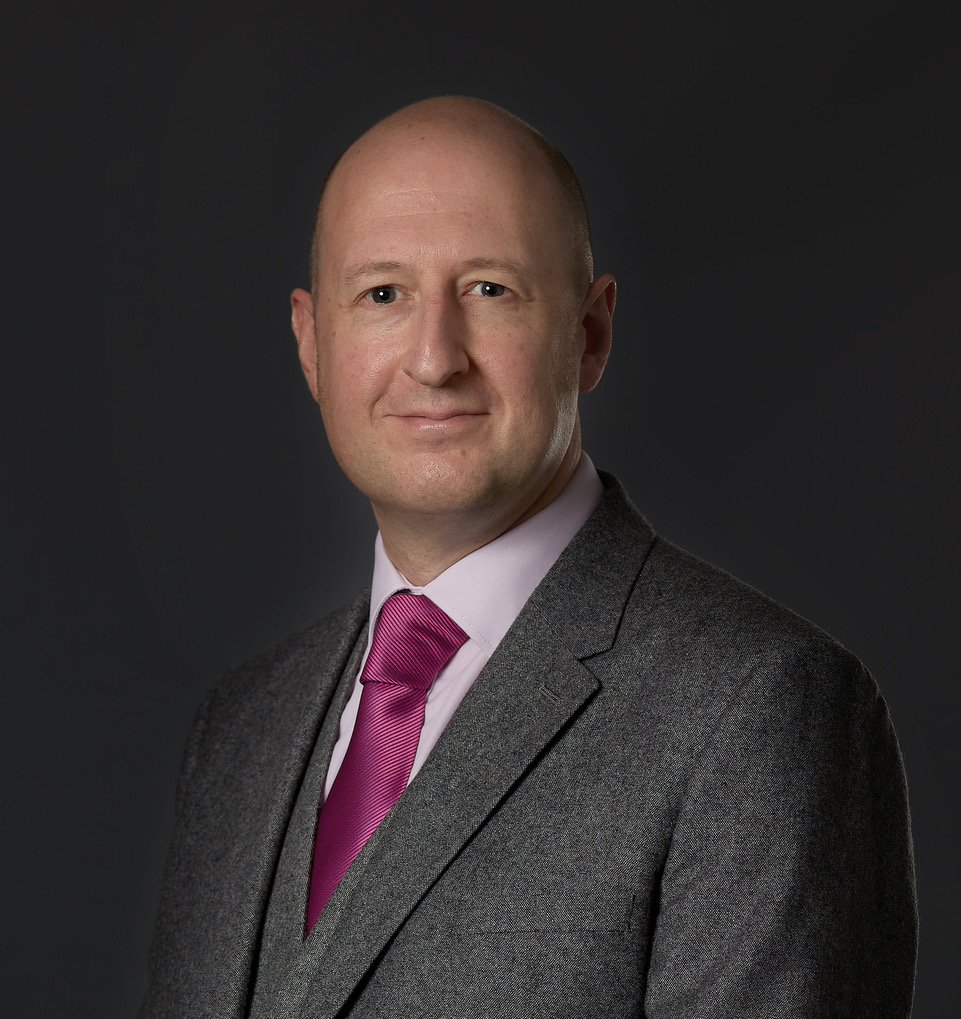
We are trying to make it easier for automation, or control engineers to take on some of those data science activities. This we can do in our ThingWorxs Analytics product that consumes the data and it goes through some of those steps that that data scientists would have to go through. It provides innovative solution templates that put data science in the hands of the domain experts.
It can look through tags to correlate which are required for the optimal prediction. Out of a hundred, or even a thousand there might be just five that can have a major impact. It then automatically goes through what is called auto machine learning, which helps pick which algorithm to run, and even starts to run through a number of scenarios to pick which algorithm or collection of algorithms gives the best output.
It is that sort of simplification, of what is a complex process, that will allow the domain experts to really extract the value that is locked up in the data collected and herald in the age of the citizen data scientist.
The author is Mike Loughran CTO for the UK and Ireland at Rockwell Automation.
About the author
Mike Loughran is CTO for the UK and Ireland at Rockwell Automation, a provider of industrial automation and information technology. He has been with the company for more than 14 years, having begun in the area of software sales and moving up the ladder to the C-suite position he now holds.
Comment on this article below or via Twitter: @IoTNow_OR @jcIoTnow
Leave a Reply